Deep Learning - CNN to Classify FashionMNIST
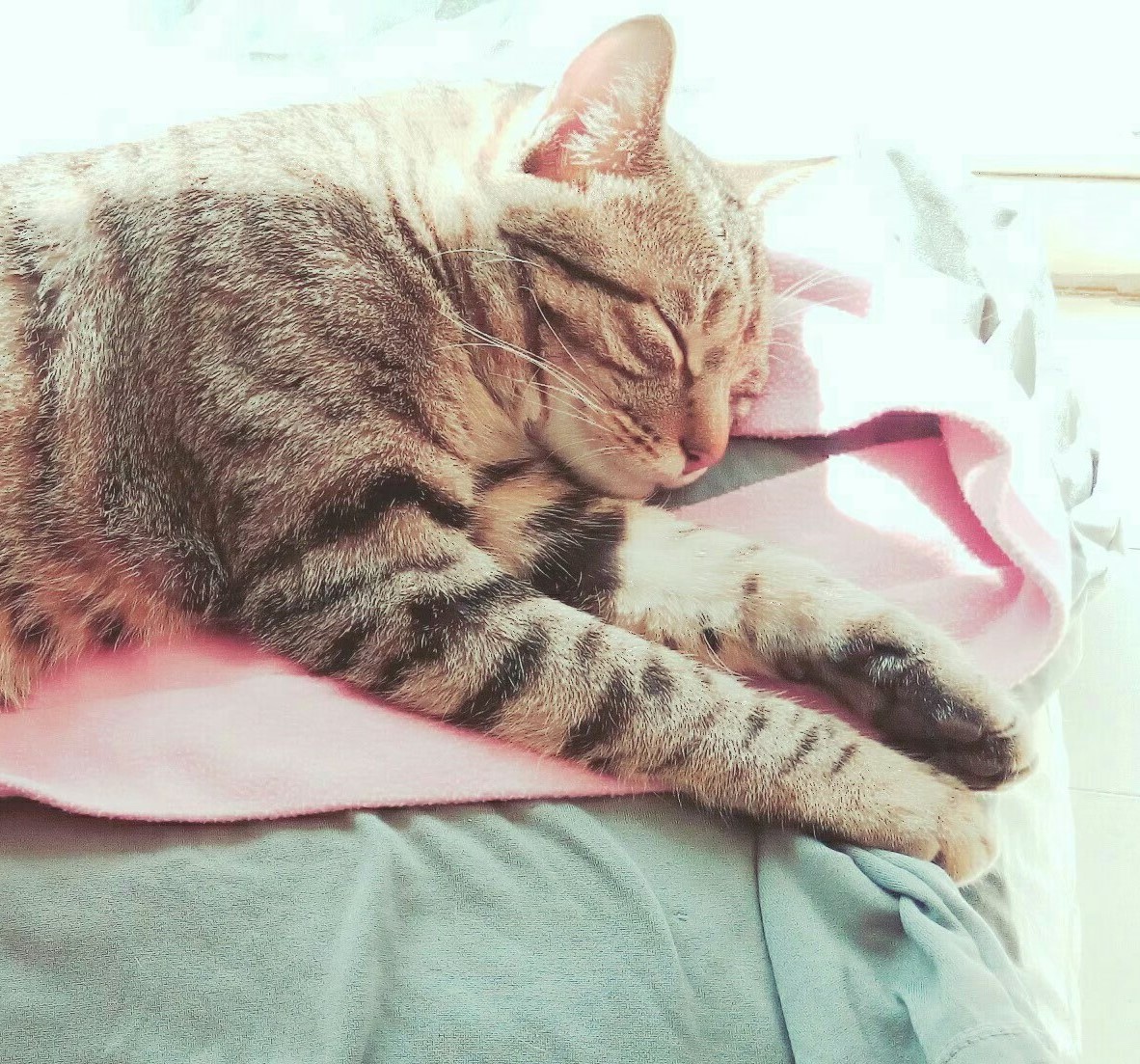
CNN to Classify FashionMNIST
利用 PyTorch 實作卷積神經網路(Convolutional Neural Network, CNN)
CNN by PyTorch
輸入資料本實驗採用含有 10
個類別的 Fashion-MNIST 數據集,每筆資料為 28x28
灰階圖像,共有 60,000
筆訓練資料和 10,000
筆測試資料
import 套件
1 | import torch |
建立 CNN 網路
詳細捲積層、池化層、全連接層、dropout 如下
1 | # 利用 CNN 於 FashionMNIST 資料 |
設定超參數
接下來會把 def train()
中的每一步逐步拆解說明
1 | def train(): |
讀檔
因為題目只有 Training Dataset,所以另外切割 20%
的資料為 Validation Dataset
可以選擇對資料做資料擴充
這裡採取了 transforms.RandomRotation
,也就是隨機角度旋轉圖片,將其轉化為 tensor 型態,然後做 normalize
1 | # def train(): |
資料切割與處理
1 | # def train(): |
宣告模型、Loss Function、Optimizer
model 為我們建立的 CNN
loss function 是 CrossEntropyLoss
採取 SGD (Stochastic Gradient Decent) 隨機梯度下降法
1 | # def train(): |
模型訓練
這次用 Min-Batch Stochastic Gradient Descent
反向傳播三板斧optimizer.zero_grad()
清除所有梯度loss.backward()
透過反向傳播獲得每個參數的梯度值optimizer.step()
透過梯度下降執行參數更新
1 | # def train(): |
計算訓練模型的準確率
1 | # def train(): |
畫出 train loss 和 validation loss
1 | # def train(): |
測試模型
1 | # def train(): |
輸出最終結果
1 | # def train(): |
main
1 | if __name__ == '__main__': |
完整程式
1 | import torch |
Reference
- 黃貞瑛老師的深度學習課程
- 許瀚丰、應名宥學長的助教輔導課程
- 吳建中同學的共同討論